Implementing a CNN with numpy
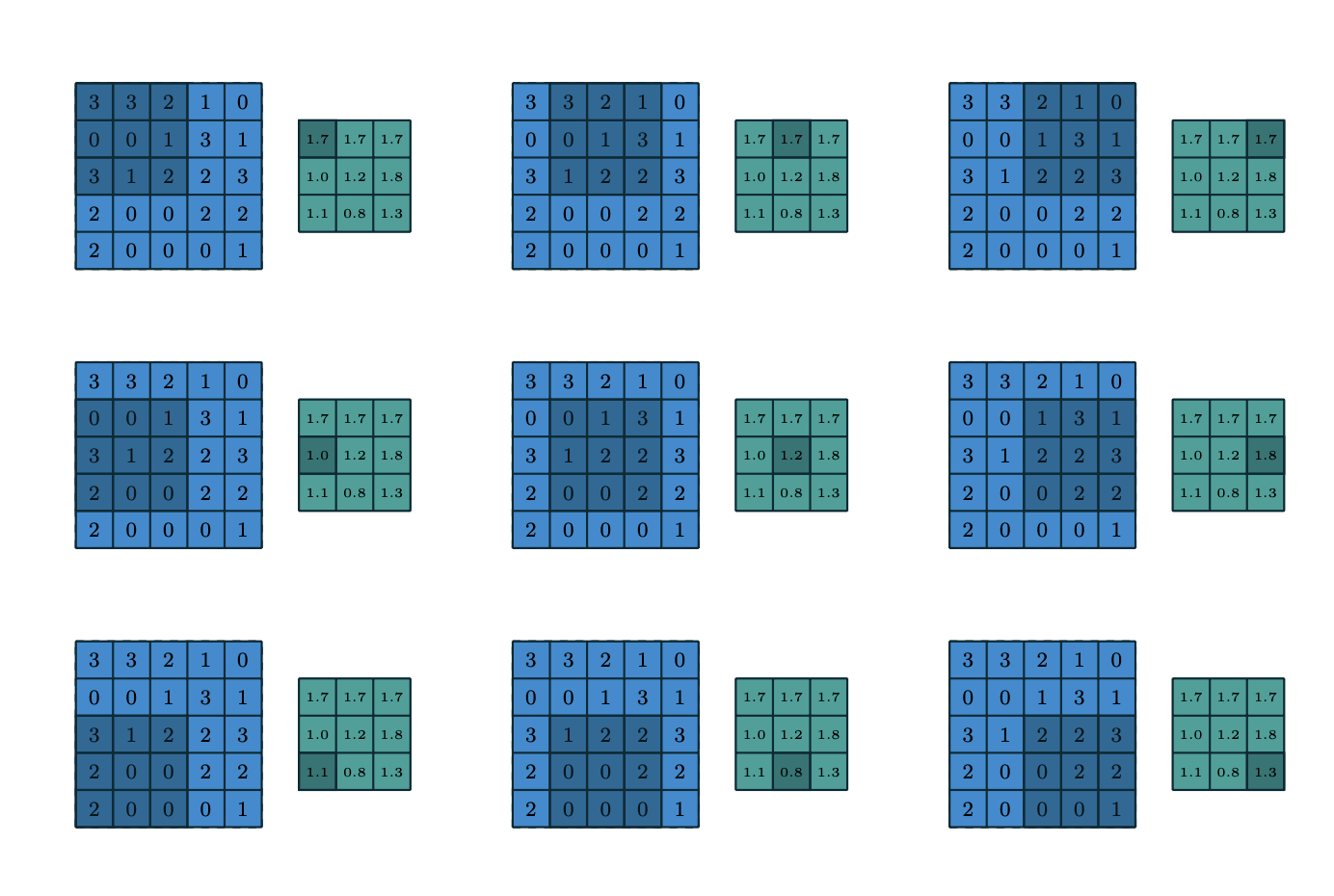
This post is a follows directly from my MLP post. In fact, both of these implementations come from the my deep learning class of 2023.
Motivation
During my deep learning class of 2023, we were asked to build from a CNN with numpy.The goal was to develop a primitive but working implementation of a CNN with the help of Numpy. This part does not contain any experimentations and was originaly coded in a jupyter notebook.
What’s in here
There are two building blocks to a convolution layer: The convolution layer and the pooling layer. This implementatin is restricted to 2d convolution (think black and white images) with a max pooling layer. Both layers make use of a ‘view’ to compute the convolution and the pooling in a local area.
Implementing the convolution layer of CNN with Numpy
import matplotlib.pyplot as plt
import seaborn as sns
import numpy as np
import random
sns.set()
class Convolution2dLayer(object):
"""
Implements a 2D convolution layer.
"""
def __init__(self, filter_size=3, stride=1, n_outchannel=32):
"""
Constructor of the Convolution2dLayer class.
Note: We assume that the input images have only a single channel.
:param filter_size: filter size to use for convolution. We assume equal height and width. (int, default 3)
:param stride: stride for convolution. (int, default 1)
:n_outchannel: number of output channels, i.e., number of filters in the layer. (int, default 32)
"""
super().__init__()
self.filter_size = filter_size
self.stride = stride
self.n_outchannel = n_outchannel
self.initialize_weights()
def initialize_weights(self):
"""
Initializes the weights of the CNN from uniform distribution(0, 1) and the biases to zeros.
"""
# self.weights is an np.array of shape (n_outchannel, filter_size, filter_size)
# We do not consider biases in this convolution layer implementation
self.weights = np.random.uniform(low=0, high=1, size=(self.n_outchannel, self.filter_size, self.filter_size))
def _get_filtersizeXfiltersize_views(self, x):
"""
Function to iterate through the input with given stride.
:param x: input of shape (batch size, 1, h, w). We assume input has only 1 channel.
for a list of inputs, h and w must be consistent across the inputs.
pass :returns: a generator that produces the current 'view' of the input and its indices given the stride
it will be a tuple of following format: ( view's row index, view's column index, view with shape (batch size, 1, filtersize, filtersize) )
"""
rows, cols = x.shape[2], x.shape[3]
# loop over rows and columns with a stride of filter_size x filter_size to get views
for i in range(0, rows, self.stride):
for j in range(0, cols, self.stride):
if i + self.filter_size - 1 < rows and j + self.filter_size - 1 < cols:
#yield...
yield i, j, x[:, :, i:i + self.filter_size, j:j + self.filter_size]
def forward(self, x):
"""
Function to forward 2d convolution.
:param x: Inputs to convolve. This may contain multiple input examples, not just one.
Note: We assume that the input images have only a single channel.
:returns: Inputs and the result of the convolution operation on the inputs stored in cache.
"""
cache = {}
# cache is a dictionary where cache["x"] stores the inputs and cache["out"] stores the outputs of the layer
cache["x"] = x
output_h = int((x.shape[2] - self.filter_size) / self.stride + 1)
output_w = int((x.shape[3] - self.filter_size) / self.stride + 1)
cache["out"] = np.zeros((x.shape[0], self.weights.shape[0], output_h, output_w))
for view_h, view_w, view in self._get_filtersizeXfiltersize_views(x):
# cache["out"][?, ?, ?, ?] = ...
cache["out"][:, :, view_h, view_w] = np.sum(self.weights * view, axis = (2,3))
return cache
def backward(self, cache, grad_output):
"""
Function to backward gradients of 2d convolution
:param cache: dictionary containing the inputs and the result of the convolution operation applied on them.
:param grad_output: gradient of the loss w.r.t. the outputs of the convolution layer.
:returns: Gradient of the loss w.r.t. the parameters of the convolution layer.
"""
# grads is an np.array containing the gradient of the loss w.r.t. the parameters in the convolution layer
# Remember to account for the number of input examples!
x = cache["x"]
grads = np.zeros(self.weights.shape)
for i in range(self.n_outchannel):
for h in range(self.filter_size):
for w in range(self.filter_size):
grads[i, h ,w] = (grad_output[:,i,:,:].squeeze()*x.squeeze()[:,h:h+grad_output.shape[2],w:w+grad_output.shape[3]]).sum()
return grads
Implementing the max-pooling layer of CNN
class MaxPooling2dLayer(object):
"""
Implements a 2D max-pooling layer.
"""
def __init__(self, filter_size=2):
"""
Constructor of the MaxPooling2dLayer class.
:param filter_size: size of filter for max-pooling. int, default 2
we assume equal height and width, and stride = height = width = filter_size
"""
super().__init__()
self.filter_size = filter_size
def _get_filtersizeXfiltersize_views(self, x):
"""
Function to iterate through the input with given stride.
:param x: input of shape (batch size, 1, h, w). We assume input has only 1 channel.
:returns: a generator that produce the current 'view' of the input with the given stride,
will be a tuple of following format: ( view's row index, view's column index, view itself with shape (batch size, 1, filtersize, filtersize) )
"""
rows, cols = x.shape[2], x.shape[3]
# loop over rows and columns with a stride of filter_size x filter_size to get non-overlappig views
for i in range(0, rows, self.filter_size):
for j in range(0, cols, self.filter_size):
if i + self.filter_size - 1 < rows and j + self.filter_size - 1 < cols:
# yield ...
yield i//self.filter_size, j//self.filter_size, x[:, :, i:i+self.filter_size, j:j+self.filter_size]
def forward(self, x):
"""
Function to forward 2dPooling.
:param x: Inputs to compute max-pooling for. This may contain multiple input examples, not just one.
Note: The input dimensions to max-pooling are the output dimensions of the convolution!
:returns: Inputs and the result of the max-pooling operation on the inputs stored in cache.
"""
# cache is a dictionary where cache["x"] stores the inputs and cache["out"] stores the outputs of the layer
cache = {}
cache["x"] = x
output_h = int(x.shape[2] / self.filter_size)
output_w = int(x.shape[3] / self.filter_size)
cache["out"] = np.zeros((x.shape[0], x.shape[1], output_h, output_w))
for view_h, view_w, view in self._get_filtersizeXfiltersize_views(x):
# cache["out"][:, :, ?, ?] = ?
cache["out"][:,:,view_h, view_w] = np.max(view, axis = (2,3))
return cache
def backward(self, cache, grad_output):
"""
Function to backward gradients of 2dPooling.
:param cache: Contains the inputs and the result of the max-pooling operation applied on them.
:param grad_output: Gradient of the loss with respect to the outputs of the max-pooling layer.
:returns: Gradient of the loss with respect to the inputs to the max-pooling layer.
"""
x = cache["x"]
grads = np.zeros_like(x)
for view_h, view_w, view in self._get_filtersizeXfiltersize_views(x):
max_vals = x[:, :, view_h*self.filter_size:(view_h+1)*self.filter_size, view_w*self.filter_size:(view_w+1)*self.filter_size].max(axis=(2, 3), keepdims=True)
max_mask = (x[:, :, view_h*self.filter_size:(view_h+1)*self.filter_size, view_w*self.filter_size:(view_w+1)*self.filter_size] == max_vals).astype(int)
grads[:, :, view_h*self.filter_size:(view_h+1)*self.filter_size, view_w*self.filter_size:(view_w+1)*self.filter_size] = max_mask * grad_output[:, :, [[view_h]], [[view_w]]]
return grads