A quick implementation of VGG16
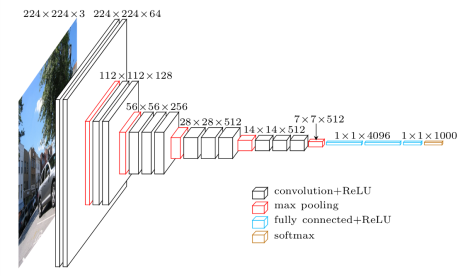
Motivation
During my deep learning class of 2023, we were asked to build a slightly different version of the VGG16 with pytorch. The program was originaly coded in a jupyter notebook.
What is the VGG16 architecture
VGG stands for Visual Geometry Group, a research group at the University of Oxford. It is a well known convolutional neural network used in image classification. Published in a 2014 paper, it gained recognition for its deep stacking of convolution layers, utilization of small filter sizes, and adherence to a uniform architecture.
Implementing the VGG16 architecture
This is simple implementation of the VGG16 model. It does not train the model, but it has some cute functions to visualize the first and last feature maps of the model.
import torch
import torch.nn as nn
import torch.nn.init as init
device = "cuda" if torch.cuda.is_available() else "cpu"
class VGG16(nn.Module):
"""This class implements the VGG-16 architecture in PyTorch"""
def __init__(self, activation_str="relu"):
"""
Constructor for the VGG16 class.
activation_str: string, default "relu"
Activation function to use.
"""
super(VGG16, self).__init__()
self.n_classes = 10
self.activation_str = activation_str
self.conv_layer_1 = nn.Conv2d(in_channels = 3, out_channels = 64, kernel_size = 3, padding = "same")
self.conv_layer_2 = nn.Conv2d(in_channels = 64, out_channels = 64, kernel_size = 3, padding = "same")
self.conv_layer_3 = nn.Conv2d(in_channels = 64, out_channels = 128, kernel_size = 3, padding = "same")
self.conv_layer_4 = nn.Conv2d(in_channels = 128, out_channels = 128, kernel_size = 3, padding = "same")
self.conv_layer_5 = nn.Conv2d(in_channels = 128, out_channels = 256, kernel_size = 3, padding = "same")
self.conv_layer_6 = nn.Conv2d(in_channels = 256, out_channels = 256, kernel_size = 3, padding = "same")
self.conv_layer_7 = nn.Conv2d(in_channels = 256, out_channels = 256, kernel_size = 3, padding = "same")
self.conv_layer_8 = nn.Conv2d(in_channels = 256, out_channels = 512, kernel_size = 3, padding = "same")
self.conv_layer_9 = nn.Conv2d(in_channels = 512, out_channels = 512, kernel_size = 3, padding = "same")
self.conv_layer_10 = nn.Conv2d(in_channels = 512, out_channels = 512, kernel_size = 3, padding = "same")
self.conv_layer_11 = nn.Conv2d(in_channels = 512, out_channels = 512, kernel_size = 3, padding = "same")
self.conv_layer_12 = nn.Conv2d(in_channels = 512, out_channels = 512, kernel_size = 3, padding = "same")
self.conv_layer_13 = nn.Conv2d(in_channels = 512, out_channels = 512, kernel_size = 3, padding = "same")
# Add 2D batch normalization after every convolutional layer
self.conv_layer_1_bn = nn.BatchNorm2d(num_features = 64)
self.conv_layer_2_bn = nn.BatchNorm2d(num_features = 64)
self.conv_layer_3_bn = nn.BatchNorm2d(num_features = 128)
self.conv_layer_4_bn = nn.BatchNorm2d(num_features = 128)
self.conv_layer_5_bn = nn.BatchNorm2d(num_features = 256)
self.conv_layer_6_bn = nn.BatchNorm2d(num_features = 256)
self.conv_layer_7_bn = nn.BatchNorm2d(num_features = 256)
self.conv_layer_8_bn = nn.BatchNorm2d(num_features = 512)
self.conv_layer_9_bn = nn.BatchNorm2d(num_features = 512)
self.conv_layer_10_bn = nn.BatchNorm2d(num_features = 512)
self.conv_layer_11_bn = nn.BatchNorm2d(num_features = 512)
self.conv_layer_12_bn = nn.BatchNorm2d(num_features = 512)
self.conv_layer_13_bn = nn.BatchNorm2d(num_features = 512)
self.max_pool_layer_1 = nn.MaxPool2d(kernel_size=2, stride = 2)
self.max_pool_layer_2 = nn.MaxPool2d(kernel_size=2, stride = 2)
self.max_pool_layer_3 = nn.MaxPool2d(kernel_size=2, stride = 2)
self.max_pool_layer_4 = nn.MaxPool2d(kernel_size=2, stride = 2)
self.max_pool_layer_5 = nn.MaxPool2d(kernel_size=2, stride = 2)
self.fc_1 = nn.Linear(in_features = 25088, out_features = 4096)
self.fc_2 = nn.Linear(in_features = 4096, out_features = 4096)
self.fc_3 = nn.Linear(in_features = 4096, out_features = self.n_classes)
# Initialize the weights of each trainable layer of the network using xavier_uniform initialization
def xavier_init(layer):
for k,v in layer.named_parameters():
if k == 'weight':
init.xavier_uniform_(v)
xavier_init(self.conv_layer_1)
xavier_init(self.conv_layer_2)
xavier_init(self.conv_layer_3)
xavier_init(self.conv_layer_4)
xavier_init(self.conv_layer_5)
xavier_init(self.conv_layer_6)
xavier_init(self.conv_layer_7)
xavier_init(self.conv_layer_8)
xavier_init(self.conv_layer_9)
xavier_init(self.conv_layer_10)
xavier_init(self.conv_layer_11)
xavier_init(self.conv_layer_12)
xavier_init(self.conv_layer_13)
xavier_init(self.fc_1)
xavier_init(self.fc_2)
xavier_init(self.fc_3)
def activation(self, input):
"""
input: Tensor
Input on which the activation is applied.
Output: Result of activation function applied on input.
E.g. if self.activation_str is "relu", return relu(input).
"""
if self.activation_str == "relu":
a = nn.ReLU()
return a(input)
elif self.activation_str == "tanh":
a = nn.Tanh()
return a(input)
else:
raise Exception("Invalid activation")
return 0
def get_first_conv_layer_filters(self):
"""
Outputs: Returns the filters in the first convolution layer.
"""
return self.conv_layer_1.weight.clone().cpu().detach().numpy()
def get_last_conv_layer_filters(self):
"""
Outputs: Returns the filters in the last convolution layer.
"""
return self.conv_layer_13.weight.clone().cpu().detach().numpy()
def forward(self, x):
"""
x: Tensor
Input to the network.
Outputs: Returns the output of the forward pass of the network.
"""
x = self.conv_layer_1(x)
x = self.conv_layer_1_bn(x)
x = self.activation(x)
x = self.conv_layer_2(x)
x = self.conv_layer_2_bn(x)
x = self.activation(x)
x = self.max_pool_layer_1(x)
x = self.conv_layer_3(x)
x = self.conv_layer_3_bn(x)
x = self.activation(x)
x = self.conv_layer_4(x)
x = self.conv_layer_4_bn(x)
x = self.activation(x)
x = self.max_pool_layer_2(x)
x = self.conv_layer_5(x)
x = self.conv_layer_5_bn(x)
x = self.activation(x)
x = self.conv_layer_6(x)
x = self.conv_layer_6_bn(x)
x = self.activation(x)
x = self.conv_layer_7(x)
x = self.conv_layer_7_bn(x)
x = self.activation(x)
x = self.max_pool_layer_3(x)
x = self.conv_layer_8(x)
x = self.conv_layer_8_bn(x)
x = self.activation(x)
x = self.conv_layer_9(x)
x = self.conv_layer_9_bn(x)
x = self.activation(x)
x = self.conv_layer_10(x)
x = self.conv_layer_10_bn(x)
x = self.activation(x)
x = self.max_pool_layer_4(x)
x = self.conv_layer_11(x)
x = self.conv_layer_11_bn(x)
x = self.activation(x)
x = self.conv_layer_12(x)
x = self.conv_layer_12_bn(x)
x = self.activation(x)
x = self.conv_layer_13(x)
x = self.conv_layer_13_bn(x)
x = self.activation(x)
x = self.max_pool_layer_5(x)
x = torch.flatten(x, start_dim = 1)
x = self.fc_1(x)
x = self.activation(x)
x = self.fc_2(x)
x = self.activation(x)
x = self.fc_3(x)
#x = self.activation(x)
softmax = nn.Softmax(dim = 1)
o = softmax(x)
return o
def last_conv(self, x):
"""
x: Tensor
Input to the network.
Outputs: Returns the output of the last convolution of the network. Useful
to visualize the last feature map.
"""
x = self.conv_layer_1(x)
x = self.conv_layer_1_bn(x)
x = self.activation(x)
x = self.conv_layer_2(x)
x = self.conv_layer_2_bn(x)
x = self.activation(x)
x = self.max_pool_layer_1(x)
x = self.conv_layer_3(x)
x = self.conv_layer_3_bn(x)
x = self.activation(x)
x = self.conv_layer_4(x)
x = self.conv_layer_4_bn(x)
x = self.activation(x)
x = self.max_pool_layer_2(x)
x = self.conv_layer_5(x)
x = self.conv_layer_5_bn(x)
x = self.activation(x)
x = self.conv_layer_6(x)
x = self.conv_layer_6_bn(x)
x = self.activation(x)
x = self.conv_layer_7(x)
x = self.conv_layer_7_bn(x)
x = self.activation(x)
x = self.max_pool_layer_3(x)
x = self.conv_layer_8(x)
x = self.conv_layer_8_bn(x)
x = self.activation(x)
x = self.conv_layer_9(x)
x = self.conv_layer_9_bn(x)
x = self.activation(x)
x = self.conv_layer_10(x)
x = self.conv_layer_10_bn(x)
x = self.activation(x)
x = self.max_pool_layer_4(x)
x = self.conv_layer_11(x)
x = self.conv_layer_11_bn(x)
x = self.activation(x)
x = self.conv_layer_12(x)
x = self.conv_layer_12_bn(x)
x = self.activation(x)
x = self.conv_layer_13(x)
x = self.conv_layer_13_bn(x)
x = self.activation(x)
x = self.max_pool_layer_5(x)
return x